The Problem With Data Lakes
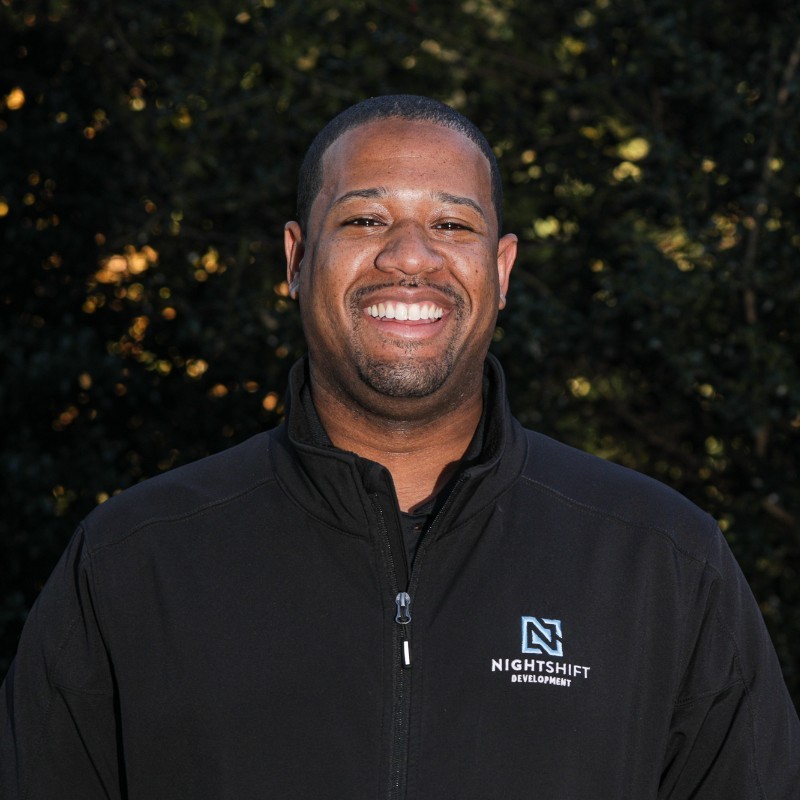
And 5 Must-Have Strategies To Make Yours Effective

As organizations become more technologically savvy and begin realizing the true value of all of the data they are collecting they will inevitably be faced with two pressing questions: “Where do I put it all?” and “Which data is important?”. At the rate that data is generated, organizations that aren’t prepared for the massive amounts of data coming their way typically fall into the trap of shoving all of their data into a data lake… Where, in most cases, data goes to die.
What is a data lake and why is it a trap?
The promise of the “data lake” was a compelling one — instead of many data silos across an organization (where data is not shared), have one consolidated repository where everyone has access to all of your organization’s data (structured and unstructured) and can get value from it. You have one location where every bit of your data lands whether that is structured transactional data or unstructured social media content where it can be analyzed later. Data lakes sound appealing — capture all of my data now in a centralized location and do the analysis later. Where they fall flat, however, is in the implementation.

The reality is that organizations that don’t have a comprehensive data strategy in place wind up building more of a data swamp. These swamps wind up being places where data is hoarded never deleted in fear of losing some analytic value, but also restrict access to a small number of individuals in fear of providing access to sensitive data to the wrong people. The dirty secret that most data lake vendors/cloud providers will never tell you is that they love when organizations end up building data swamps — they get paid more the more you hoard data.
5 Must-Have Strategies For Data Lakes
Data Lakes don’t have to be all bad though. With the right strategies in place when your data lake is implemented, your organization can realize the true value and save money at the same time.
Here are the 5 must-have strategies for Data Lakes:
- Data Discovery Strategy
One of the most important strategies for any data lake is a data discoverability strategy. If no one knows what data is available and where that data lives it will be hard for anyone to get value from it. One of the first things you should have in place when setting up your data lake is a data catalog. The data catalog should identify the source of the data, who owns it, what the data access rules are, how frequently it is updated when it was last updated, and provide a description of what exactly is in it, and in the cases of structured data, a data schema and dictionary. A comprehensive data discovery strategy helps ensure that all data your organization collects can be consumed by the right individuals. - Access Control Strategy
In the age of GDPR, big data, and the every other day hack, consumers and regulators are becoming much savvier about data privacy and data security issues. This means your organization is responsible for ensuring that principles of least privilege are implemented when it comes to the most sensitive data in your organization. At the same time, you want to ensure that data is as accessible as possible to those that can get value from it. Access control strategies should potentially factor in things like row or even cell-level security for the most sensitive data, and policies for validation of access to data. These access control policies should also extend beyond the data lake and into downstream analytics solutions. A comprehensive access control strategy will ensure data is shared as widely as possible while protecting your organization's most sensitive data. - Data Lifecycle Strategy
In the era of the data lake, there is a common misconception that all data needs to be kept forever to ensure nothing is ever missed. The reality is, most data has a shelf-life. Depending on the industry you’re in and the specific data you’re collecting, it’s highly likely that there is some decay function that can be applied to the value of a given data source as time progresses. Each dataset your organization collects should be analyzed to determine the relative value of keeping that data around over time. In some cases you can get rid of it entirely, in many others you can roll that data up and keep aggregate statistics around for analytic value rather than the full source data. There should be relatively few datasets that actually need to stay around in their entirety forever. When an effective data lifecycle strategy is implemented, organizations can save costs on their data lakes and ensure they aren’t hoarding data that results in that lake becoming a swamp. - Real-Time Processing Strategy
One of the most effective ways to tame your data lake is to perform some data processing at the edge. This means as data is streaming in it is processed in some way and consumable insights are produced on the fly. In some cases, once those insights are produced, you no longer need to keep the source data around. You can additionally apply machine learning to categorize or add filtering rules to determine what needs to be stored in your data lake as it streams through. Not keeping that data around could mean some loss of historical analysis, so it is important to ensure your real-time processing strategy also factors in your longer-term data analytics goals. With a real-time processing strategy in place, organizations can keep data organized and minimize what is kept in the data lake resulting in significant cost savings. - Data Analytics & Democratization Strategy
The ultimate goal for data lakes is deferring analysis until later when you’re unsure about the value of the data you’re collecting. Collecting data and not having a solid data analytics strategy for your organization defeats the purpose. It’s important to have a plan for how that data might be analyzed later and having tools in place to help democratize the access to data across your organization as much as possible. This means having the right tools for your data scientists all the way through to your non-technical domain experts. You may not know whether data you’re collecting has value right at the start, but you should at least have a plan for how you will analyze it later to determine if there is value. A data analytics & democratization strategy that considers all potential consumers of your data will set your organization up for success.
Wrapping Up
Data Lakes come with a tremendous promise of providing organizations a way to defer the analysis of data while making sure they collect everything they need. When improperly implemented data lakes can actually result in significant costs and not a lot of return on investment. Having the 5 critical strategies identified above in place can help ensure your organization avoids the traps of data lakes and sets you up for longer-term success.
At Night Shift Development, we’re on a mission to democratize data analytics and focus heavily on helping organizations make sense of their data. If these strategies seem daunting, or you’re interested in learning more about how we can help you define and implement a comprehensive set of strategies, please reach out to schedule a free consultation!